|
  |
 |
 |
Mahalanobis Encodings for Visual Categorization |
|
|
|
|
Nowadays, the design of the representation of images is one of the most crucial factors in the performance of visual categorization. A common pipeline employed in most recent researches for obtaining an image representation consists of two steps: the encoding step and the pooling step. This study introduces the Mahalanobis metric to
two popular image patch encoding modules, Histogram Encoding
and Fisher Encoding.
|
|
|
|
|
|
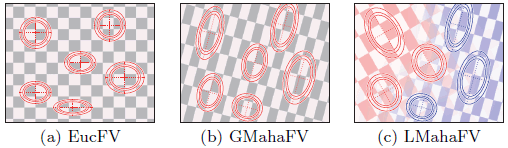 |
|
|
Figure 1.Visual Words on Different Metric Spaces.In this example, six visual words are in the codebook with different
metrics. (a) EucFV uses visual words on the Euclidean metric. (b) GMahaFV method replaces the Euclidean metric to a single Mahalanobis metric. (c) LMahaFV method divides the image patch space softly into two subsets each of which is on a different metric, and three visual words are given to each of the two subsets. |
|
|
|
|
|
References |
|
|
Tomoki Matsuzawa, Raissa Relator, Wataru Takei, Shinichiro Omachi and Tsuyoshi Kato, Mahalanobis Encodings for Visual Categorization, IPSJ Transactions on Computer Vision and Applications, vol x, pp.xx--xx, 2015, Jul, presented at MIRU2015 as Oral Presentation. MIRU2015 student award. |
|
|
|
|
|