|
  |
 |
 |
Efficient Metric Learning - Bregman Divergence Regularized Machine |
|
|
|
|
We consider using the Mahalanobis distance for the task. Classically, the inverse of a covariance matrix has been chosen as the Mahalanobis matrix, a parameter of the Mahalanobis distance. Modern studies often employ machine learning algorithms called metric learning to determine the Mahalanobis matrix so that the distance is more discriminative, although they resort to eigen-decomposition requiring heavy computation. This paper presents a new metric learning algorithm that finds discriminative Mahalanobis matrices efficiently without eigen-decomposition, and shows promising experimental results on real-world face-image datasets.
|
|
|
|
|
|
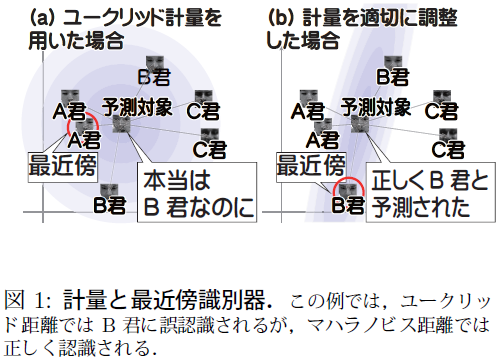 |
|
|
|
|
|
|
|
|
References |
|
|
Tsuyoshi Kato, Wataru Takei, Shinichiro Omachi "A Discriminative Metric Learning Algorithm for Face Recognition"IPSJ Transactions on Computer Vision and Applications, vol 5, pp.85--89, presented at MIRU2013 as Oral Presentation. |
|
|
|
|
|