|
  |
 |
 |
Fuzzy Subspace Clustering and Outlier Detection |
|
|
|
|
Anomaly detection has several practical applications in different areas, including intrusion detection, image processing, and behavior analysis among others. Several approaches have been developed for this task such as detection by classification, nearest neighbor approach, and clustering. This paper proposes alternative clustering algorithms for the task of anomaly detection. By employing a weighted kernel extension of the least squares fitting of linear manifolds, we develop fuzzy clustering algorithms for kernel manifolds. Experimental results show that the proposed algorithms achieve promising performances compared to hard clustering techniques.
|
|
|
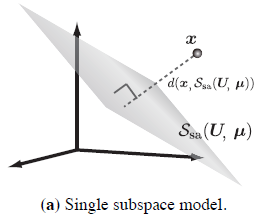 |
|
|
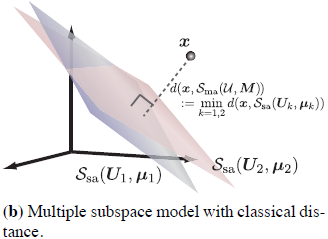 |
|
|
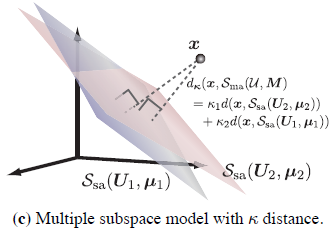 |
|
|
Fig. 1 Normal class models. In this study, the normal class is modeled by a set of points. Classically, the anomalism of an input data point is examined
with the distance to its projection onto the point set. Figure (a) describes a model that uses a single affine subspace. In (b), the
normal class model is given by the union of two affine subspace. The classical distance to the set is the square
Euclidean distance to the nearest subspace. In this study, we introduce the κ-distance that is the linear combination of the distances with weights κ1 and κ2,
as in (c) . |
|
|
|
|
|
References |
|
|
Raissa Relator, Tsuyoshi Kato, Takuma Tomaru, Naoya Ohta, Fuzzy Multiple Subspace Fitting for Anomaly Detection, IEICE Transactions on Information & Systems, Vol.Exx-D,No.x,pp.-, accepted. |
|
|
|
|
|