|
software
  |
 |
 |
Deep Learning for virus detection from TEM images |
|
|
|
|
A new computational method for the detection of virus particles in transmission electron microscopy (TEM) images is
presented. Our approach is to use a convolutional neural network that transforms a TEM image to a probabilistic map that
indicates where virus particles exist in the image. Our proposed approach automatically and simultaneously learns both
discriminative features and classifier for virus particle detection by machine learning, in contrast to existing methods that
are based on handcrafted features that yield many false positives and require several postprocessing steps. The detection
performance of the proposed method was assessed against a dataset of TEM images containing feline calicivirus particles
and compared with several existing detection methods, and the state-of-the-art performance of the developed method for
detecting virus was demonstrated. Since our method is based on supervised learning that requires both the input images
and their corresponding annotations, it is basically used for detection of already-known viruses. However, the method is
highly flexible, and the convolutional networks can adapt themselves to any virus particles by learning automatically from
an annotated dataset. |
|
|
|
|
|
19-viruscnn1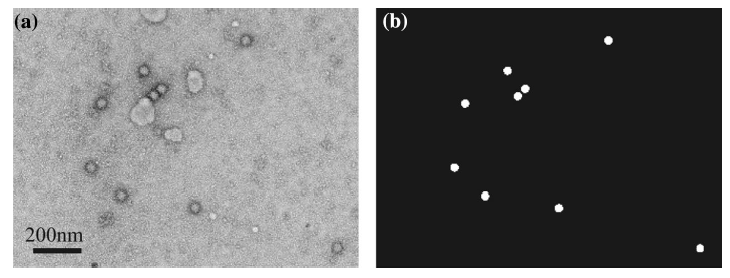 |
|
|
The problem settings of
our study. (a) Input image (grayscale), (b) ground truth manually
created by human experts
(binary). The input image
contains multiple viral particles,
debris, and a noisy background.
The contrast of the image is
varied at some locations. |
|
|
|
|
|
19-viruscnn1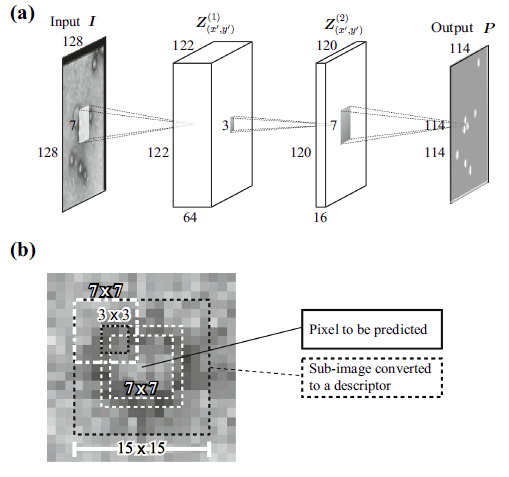 |
|
|
(a) Network structure of the proposed FCN. (b) The size
of each filter. The filters were designed in this study so that the final
prediction for a pixel in the input image depends on a 15 × 15 subimage
that covers the target particle and the surrounding region in the
input image. |
|
|
|
|
|
|
|
|
References |
|
|
Eisuke Ito, Takaaki Sato, Daisuke Sano, Etsuko Utagawa, Tsuyoshi Kato, Virus Particle Detection by Convolutional Neural Network in Transmission Electron Microscopy Images, Food and Environmental Virology, 10(2), 201-208. doi: 10.1007/s12560-018-9335-7. |
|
|
|
|
|
|
|
|
|
|
|
|
|
|