|
software
  |
 |
 |
重みつきカーネル正準相関分析とリガンド予測への応用 |
|
|
|
|
|
Protein-ligand interaction prediction plays an important role in drug design and discovery. However, wet lab procedures are inherently time consuming and expensive due to the vast number of candidate compounds and target genes. Hence, computational approaches becames imperative and have become popular due to their promising results and practicality. Such methods require high accuracy and precision outputs for them to be useful, thus, the problem of devising such an algorithm remains very challenging. In this paper, we propose an algorithm employing both support vector machine (SVM) and an extension of canonical correlation analysis (CCA). Following assumptions of recent chemogenomic approaches, we explore the effects of incorporating bias on similarity of compounds. We introduce kernel weighted CCA as a means of unconvering any underlying relationship between similarity of ligands and known ligands of target proteins. Experimental results indicate statistically significant improvement in the area under the ROC curve and F-measure values obtained as opposed to those gathered when only SVM, or SVM with kernel CCA is employed, which translates to better quality of prediction. |
|
|
|
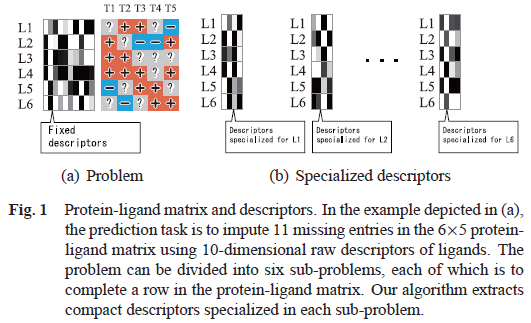 |
|
|
|
|
|
|
|
|
文献 |
|
|
Raissa Relator, Tsuyoshi Kato, Richard Lemence, Improved protein-ligand prediction using kernel weighted canonical correlation analysis, IPSJ Transaction on Bioinformatics, vol. 6, pp.18--28, June, 2013. [pdf][bibtex] |
|
|
|
|
|